Participant recruitment strategies in research
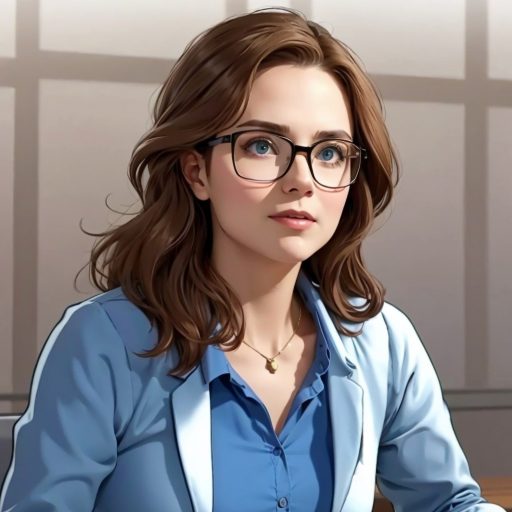
Dr Lynette Pretorius
Contact details
Dr Lynette Pretorius is an award-winning educator and researcher specialising in doctoral education, academic identity, student wellbeing, AI literacy, autoethnography, and research skills development.
The way researchers select their participants impacts the validity and reliability of their findings, making participant recruitment one of the most crucial steps in the research process. But how do researchers go about this task? What strategies do they use to ensure their sample accurately reflects the broader population or the group they are investigating? Let’s explore some common participant recruitment strategies, breaking down their strengths, weaknesses, and best use cases. This post will cover six key sampling techniques: convenience sampling, purposive sampling, snowball sampling, random sampling, stratified sampling, and quota sampling.
Convenience Sampling
Convenience sampling, as the name implies, revolves around ease of access and availability. This method involves selecting participants who are nearby, easily accessible, and willing to take part in the study. It’s a go-to choice for researchers when they need to collect data quickly and with minimal effort. Instead of engaging in time-consuming and resource-intensive processes to identify and recruit participants, convenience sampling allows researchers to gather data from those who happen to be in the right place at the right time, or who meet the study’s basic criteria and are easy to contact.
One of the most notable benefits of convenience sampling is its speed and cost-effectiveness. Since participants are easy to reach, researchers can save both time and resources that would otherwise be spent on recruitment strategies, travel, or extensive outreach. For example, if you’re studying employee engagement in the workplace, you might simply survey your colleagues, since they are readily available and meet the general criteria of being employees. You don’t need to look far or conduct an elaborate recruitment process. This ease of implementation is especially valuable when dealing with limited budgets or tight deadlines. Convenience sampling also simplifies logistics, as researchers don’t need to source participants from outside their immediate environment, which can be particularly helpful in the initial stages of research where the primary goal is to test ideas or gather preliminary data.
Despite its practicality, convenience sampling carries a significant risk of bias. Since the sample is drawn from a pool of easily accessible participants, it may not reflect the diversity of the broader population. This lack of representation can lead to skewed results, limiting the generalisability of the study’s conclusions. Moreover, convenience sampling often captures a very specific subset of individuals: those who are willing to participate. People who are available and motivated to take part may differ significantly from those who are harder to reach, potentially introducing a self-selection bias. This means that the participants in your study might share certain characteristics that make them different from the larger group you’re trying to understand, thereby limiting the accuracy and breadth of the findings.
Convenience sampling is best suited for exploratory research, pilot studies, or projects where time and resources are constrained. It’s a practical method when the research goal is to test hypotheses, gather preliminary data, or explore an emerging field. However, for studies where generalising findings to a larger population is critical, convenience sampling is not recommended. In these cases, a more representative sampling method, such as random or stratified sampling, would yield more reliable and valid results.
Purposive Sampling
Purposive sampling, also known as purposeful sampling, is a strategically driven approach to participant selection, designed to align closely with the objectives of the research. Purposive sampling involves the deliberate selection of individuals who possess specific characteristics, knowledge, or experiences that are directly relevant to the study’s focus. The intention here is not to gather a wide, diverse group of participants, but to choose individuals whose particular insights can provide depth and richness to the data. In purposive sampling, researchers carefully define the criteria for inclusion, selecting participants based on how well they fit the study’s needs. This targeted approach guarantees that the participants are not only suitable but also capable of offering the kind of focused and contextually relevant information that the research seeks to uncover.
The primary strength of purposive sampling lies in its efficiency and precision. By handpicking participants based on specific criteria, researchers can ensure that every individual involved in the study has a direct connection to the research topic, which enhances the quality of the data collected. For instance, if a researcher is investigating the experiences of people recovering from cancer, they would purposefully select participants who have undergone cancer treatment, ensuring that the data collected is directly relevant to the research question. This method is especially useful in qualitative research, where the goal is often to gain a deeper understanding of a particular phenomenon rather than to generalise findings to a larger population. Moreover, purposive sampling is often more practical when working with small or hard-to-reach populations. In studies involving niche groups, such as people with rare medical conditions or members of specific subcultures, purposive sampling enables researchers to focus on finding individuals who meet the study’s strict criteria, bypassing the need for broader recruitment efforts that may yield less relevant participants.
While purposive sampling offers many advantages in terms of relevance and efficiency, it also comes with inherent limitations, the most significant of which is the risk of selection bias. Since participants are chosen subjectively by the researcher, there is always the potential for bias in the selection process. The researcher’s choices may be influenced by preconceived notions about who would provide the most useful data, which could result in an unbalanced or unrepresentative sample. Since the sample is intentionally selective, it does not provide an accurate cross-section of a broader group. As a result, purposive sampling is not ideal for studies where broad generalisability is a key objective.
Purposive sampling is most commonly employed in qualitative research, where the goal is to explore specific themes, experiences, or phenomena in great detail. It is particularly useful when researchers are investigating a clearly defined group or phenomenon, such as in case studies, ethnographic research, or studies focusing on specialised areas like mental health, education, or organisational behaviour. Additionally, purposive sampling is often used in evaluation research, where the goal is to assess a programme, policy, or intervention. By focusing on individuals with firsthand experience, researchers can gather detailed feedback that is crucial for evaluating the effectiveness of the intervention.
Snowball Sampling
Snowball sampling is a participant recruitment method that relies heavily on social networks and personal referrals to build a sample. The process begins with a small group of initial participants who are chosen based on their relevance to the study. These participants are then asked to refer others they know who meet the study’s criteria, who in turn refer more people, and so on, creating a snowball effect. Over time, the sample grows organically, expanding through connections within a specific community or network.
This method is especially useful when researchers are working with hard-to-reach populations. These might include people in marginalised groups, individuals involved in illegal activities, or those with experiences that are not easily accessible through conventional recruitment methods, such as people who have experienced homelessness or are part of underground subcultures. In many cases, people within these groups may not want to reveal their identities to researchers, especially if their involvement in the group is sensitive or stigmatised. However, through personal referrals from trusted peers, they may be more likely to participate. The trust established between members of the community can make them more comfortable with sharing their experiences, allowing researchers to collect rich, authentic data from participants who would otherwise be unreachable. Snowball sampling can also be highly cost-efficient and flexible.
Despite its advantages, snowball sampling has several potential drawbacks, the most notable being the risk of bias. Since participants are recruited through personal networks, the sample is often restricted to people who are socially connected, which can limit the diversity of the sample. This lack of diversity can skew the results, making it difficult to generalise findings to the broader population. Moreover, snowball sampling can create a chain of referrals that is disproportionately shaped by the initial participants. If the first few participants are not representative of the population being studied, their referrals may perpetuate this imbalance, further reducing the sample’s representativeness. Another challenge is the difficulty in controlling the sample size. Since snowball sampling relies on personal referrals, the growth of the sample can be unpredictable. In some cases, the “snowball” may gather momentum quickly, leading to a large, varied participant pool. In other instances, recruitment may stall if participants are unwilling or unable to refer others, resulting in a sample that is too small to draw meaningful conclusions.
Given its strengths and limitations, snowball sampling is most effective in studies where recruiting participants through traditional methods would be difficult or impractical. It is particularly well-suited for research involving rare populations, sensitive topics, or hidden communities where members may be reluctant to come forward on their own. This method is also useful in qualitative research, where the goal is to collect in-depth, nuanced data from a specific group rather than to achieve broad generalisability. In exploratory research, snowball sampling can help researchers generate preliminary data about populations that are otherwise difficult to access. It allows for a gradual expansion of the sample, giving researchers the flexibility to adjust their recruitment strategy based on the data collected. However, because of the potential for bias, snowball sampling is generally not recommended for studies that require representative samples or where generalisability to the broader population is a primary concern.
Random Sampling
Random sampling, as the name suggests, is a method where each individual in the population has an equal chance of being selected, which makes the process akin to drawing names out of a hat. By giving every person an equal opportunity to be included, random sampling maximises the likelihood that the sample will accurately represent the broader population. A simple example would be assigning numbers to everyone in a population and using a random number generator to pick participants. This approach minimises bias and maximises the likelihood that the sample is representative. This quality is what makes random sampling a preferred choice in large-scale surveys and experimental research, where the goal is to ensure that the findings can be applied to a larger group.
One of the most notable strengths of random sampling is its ability to provide high external validity. Since the method does not favour any particular subset of the population, the findings from a study using random sampling are more likely to be generalisable—meaning that they can be applied to the wider population with a greater degree of confidence. Another key benefit is the reduction of systematic bias. In other sampling methods, certain individuals or groups may be over-represented due to researcher influence or convenience. With random sampling, this risk is minimised because the selection process is completely unbiased. The random nature of this method ensures that personal preferences, biases, or logistical factors do not affect who is chosen for the study.
Despite its many advantages, random sampling can be challenging to implement, particularly in studies with large populations. Some of the main drawbacks are the time and cost involved. To conduct random sampling on a large scale, researchers need access to a complete and up-to-date list of the population from which they’re drawing their sample. In some cases, obtaining such a list can be difficult or impossible, especially when working with fragmented or hard-to-reach populations. Additionally, there can be significant logistical hurdles. In small populations, random sampling may be fairly straightforward, but when dealing with larger populations, coordinating a random selection process can become complex. This can involve significant costs, not just in terms of the initial recruitment of participants, but also in terms of travel, communication, and follow-up procedures.
Given the costs and logistical challenges, random sampling is best suited for large quantitative studies, particularly those where generalisability is the primary goal. If the research is designed to draw conclusions about the broader population, such as in public health research, market research, or large-scale sociological studies, random sampling is ideal because it provides the most unbiased and representative data possible. In cases where time and budget constraints are more pressing, or where the research is exploratory rather than aiming for population-level generalisability, other sampling methods (such as convenience or purposive sampling) might be more appropriate.
Stratified Sampling
Stratified sampling is a method used by researchers to ensure that their sample accurately reflects the diversity of the population by focusing on key subgroups, or “strata.” The basic idea is that the population is divided into distinct groups based on important characteristics such as age, gender, income level, education, or ethnicity. Once these groups are defined, participants are then randomly selected from each stratum. This approach allows researchers to ensure that the sample mirrors the proportions of these subgroups in the overall population, leading to more precise and reliable findings. For example, if a population consists of 40% males, 55% females, and 5% transgender people, the researcher ensures that the sample has the same proportional representation. This method is particularly effective in studies where the population consists of individuals with varying characteristics that could influence the outcome of the study. By ensuring that all relevant subgroups are proportionally represented, stratified sampling helps researchers avoid over-representing or under-representing certain groups.
One of the main strengths of stratified sampling is its ability to produce a highly representative sample of the population. By ensuring that each subgroup is properly represented, this method increases the precision of the results, which in turn improves the reliability of the study’s findings. This is especially important in research where differences between subgroups are a key focus. Moreover, by dividing the population into strata and then randomly selecting participants from each group, stratified sampling ensures a more balanced and accurate representation, which minimises the risk of sampling errors. Finally, the ability to analyse subgroup differences is a key advantage of stratified sampling, particularly in fields like sociology, economics, and public health, where understanding these differences is critical.
While stratified sampling offers many advantages, it does come with certain challenges, particularly in terms of the time and resources required to implement it. One of the most time-consuming aspects of this method is the need to define and organise the strata before selecting participants. Researchers must have a clear understanding of which characteristics are most relevant to the study and must have detailed information about the population to create the strata. Furthermore, in some cases, this information may not be readily available, or the population may be too complex to neatly divide into well-defined strata. Stratified sampling can also be more logistically complicated than simpler methods like convenience sampling. Researchers need to ensure that they have enough participants in each stratum to allow for meaningful analysis, which can require more recruitment efforts. If some strata are smaller or harder to reach, the researcher may need to put in extra effort to find participants from those groups, increasing both time and costs.
Given its ability to provide a highly representative sample, stratified sampling is best used in studies where representation across key subgroups is critical. It is particularly useful when researchers are interested in analysing differences between subgroups, such as age, income, or geographic location. Stratified sampling is also valuable in demographic studies, where the goal is often to understand the characteristics of various subgroups within a population.
Quota Sampling
Quota sampling is a sampling method that shares certain goals with stratified sampling, particularly the aim of capturing diversity across specific subgroups. However, the fundamental difference lies in how the sample is selected. While stratified sampling relies on random selection from each subgroup, quota sampling allows researchers to directly control who is recruited by actively seeking participants to fill predefined quotas based on certain characteristics, such as age, gender, education level, or income. Once the quota for each subgroup is filled, no further participants from that group are recruited, ensuring that the final sample meets the predetermined criteria for representation.
One of the main advantages of quota sampling is that it guarantees the inclusion of specific subgroups in the sample. By setting quotas for each group, the researcher ensures that the final sample reflects the desired characteristics or proportions, which is particularly important when the goal of the research is to compare different groups. Another key benefit of quota sampling is its efficiency. Since the researcher can directly seek out participants who meet the required criteria, the process can be completed more quickly and at a lower cost than methods like stratified sampling. Moreover, quota sampling offers a greater degree of control over the composition of the sample. The researcher can adjust the quotas based on the needs of the study, ensuring that specific groups are represented according to the study’s objectives.
Despite its advantages, quota sampling also has several limitations, the most significant of which is the potential for bias. Since participants are not selected randomly, there is a risk that the sample may not accurately represent the broader population, even if the quotas are met. The recruitment process is subjective, as it relies on the researcher’s judgement and outreach methods, which can introduce selection bias. This lack of randomisation means that the results from a quota sample may not be generalisable to the larger population, especially if certain characteristics or perspectives are overlooked during recruitment. Additionally, quota sampling can lead to incomplete representation within each subgroup. While the researcher may set quotas based on broad characteristics like age or gender, other important factors may not be considered. This can result in a sample that, while meeting the quota criteria, lacks internal diversity within the subgroups, which can limit the depth and richness of the data collected. Another challenge with quota sampling is that it requires detailed knowledge of the population beforehand. The researcher must have a clear understanding of the proportions of different groups within the population to set accurate quotas. This can be difficult if reliable demographic data is not available, or if the population is highly fragmented or diverse in ways that are not easily captured by simple quotas.
Quota sampling is best suited for studies where the primary goal is to compare specific groups or ensure representation across key subgroups. It is commonly used in market research, opinion polling, and social research, where researchers need to gather data quickly and cost-effectively while ensuring that certain groups are represented. This method is also useful in studies where strict randomisation is not feasible or necessary. For example, in research involving focus groups or interviews, where the goal is to gather in-depth insights from specific subgroups, quota sampling allows the researcher to select participants who fit the desired profile without the logistical complexities of random selection.
You can also learn more about research designs and methods by watching the videos below.
Questions to ponder
How do different sampling methods influence the validity of research findings?
Can convenience sampling ever be justified in large-scale research?
In what scenarios might snowball sampling offer a better solution than random sampling?
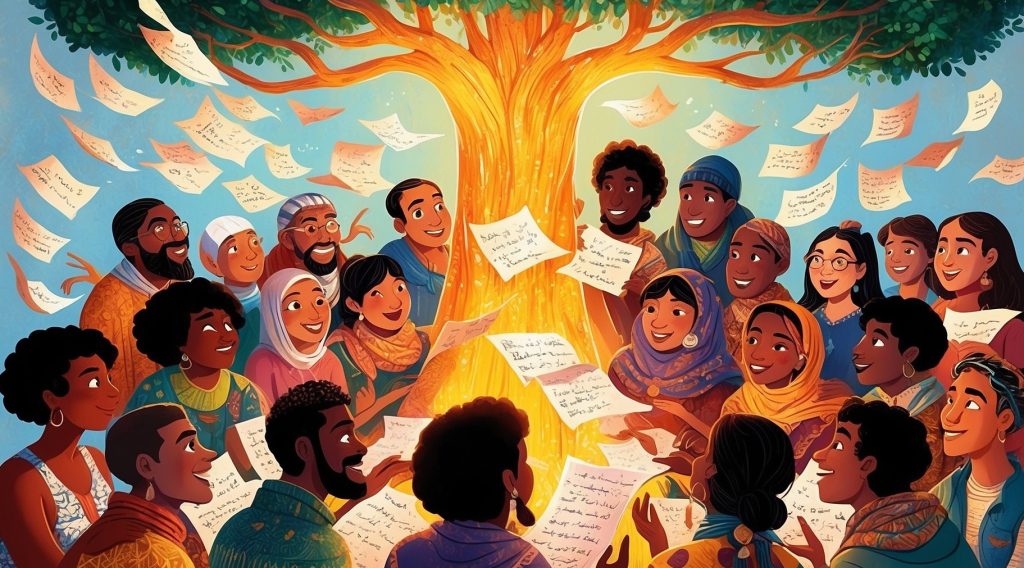